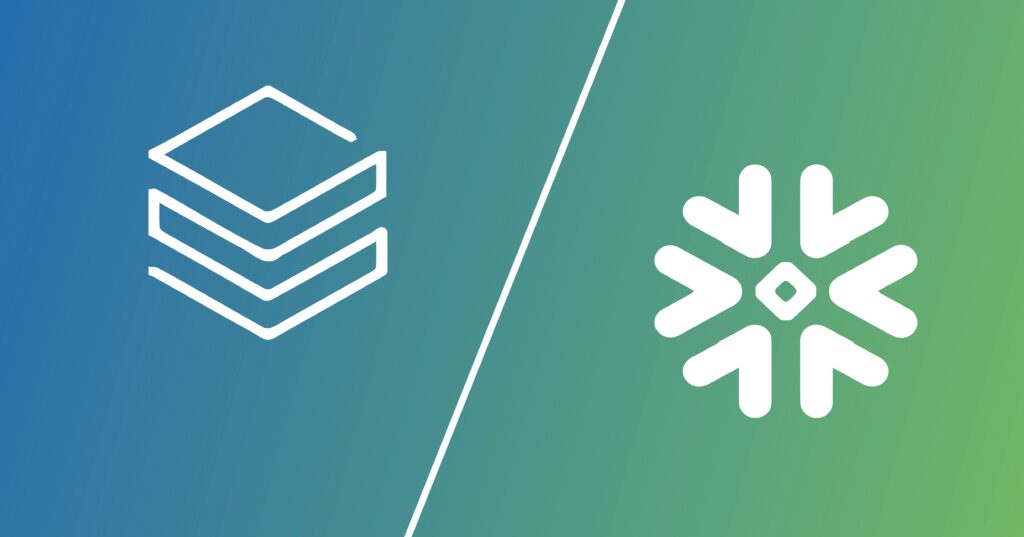
SnowFlake vs Databricks
Snowflake and Databricks are leading cloud data platforms, but how do you choose the right one for your needs? Both have different strengths, though they sometime overlap. I listed out some bullet points to highlight the differences between the two.
๐ย ๐๐ง๐จ๐ฐ๐๐ฅ๐๐ค๐
โ๏ธ ๐๐๐ญ๐ฎ๐ซ๐: Snowflake operates as a cloud-native data warehouse-as-a-service, streamlining data storage and management without the need for complex infrastructure setup.
โ๏ธ ๐๐ญ๐ซ๐๐ง๐ ๐ญ๐ก๐ฌ: It provides robust ELT (Extract, Load, Transform) capabilities primarily through its COPY command, enabling efficient data loading. Snowflake offers dedicated schema and file object definitions, enhancing data organization and accessibility.
โ๏ธย ๐
๐ฅ๐๐ฑ๐ข๐๐ข๐ฅ๐ข๐ญ๐ฒ: One of its standout features is the ability to create multiple independent compute clusters that can operate on a single data copy. This flexibility allows for enhanced resource allocation based on varying workloads.
โ๏ธ ๐๐๐ญ๐ ๐๐ง๐ ๐ข๐ง๐๐๐ซ๐ข๐ง๐ : While Snowflake primarily adopts an ELT approach, it seamlessly integrates with popular third-party ETL tools such as Fivetran, Talend, and supports DBT installation. This integration makes it a versatile choice for organizations looking to leverage existing tools.
๐ ๐๐๐ญ๐๐๐ซ๐ข๐๐ค๐ฌ
๐งฑ ๐๐๐ญ๐ฎ๐ซ๐: Databricks is a unified data analytics platform designed for cloud-native data engineering, machine learning, and business intelligence.
๐งฑย ๐๐จ๐ซ๐: Databricks is fundamentally built around processing power, with native support for Apache Spark, making it an exceptional platform for ETL tasks. This integration allows users to perform complex data transformations efficiently.
๐งฑ ๐๐ญ๐ซ๐๐ง๐ ๐ญ๐ก๐ฌ: is a data analytics platform for big data processing and machine learning, handling structured, semi-structured, and unstructured data using Apache Spark and multiple languages like Python, SQL, and Scala.
๐งฑ ๐๐ญ๐จ๐ซ๐๐ ๐: It utilizes a ‘Data Lakehouse’ architecture, which combines the features of a data lake with the ability to run SQL queries. This model is gaining traction as organizations seek to leverage both structured and unstructured data in a unified framework.
๐ ๐๐๐ฒ ๐๐๐ค๐๐๐ฐ๐๐ฒ๐ฌ
โ
๐๐ข๐ฌ๐ญ๐ข๐ง๐๐ญ ๐๐๐๐๐ฌ: Both Snowflake and Databricks excel in their respective areas, addressing different data management requirements.
โ
๐๐ง๐จ๐ฐ๐๐ฅ๐๐ค๐โ๐ฌ ๐๐๐๐๐ฅ ๐๐ฌ๐ ๐๐๐ฌ๐: If you are equipped with established ETL tools like Fivetran, Talend, or Tibco, Snowflake could be the perfect choice. It efficiently manages the complexities of database infrastructure, including partitioning, scalability, and indexing.
โ
๐๐๐ญ๐๐๐ซ๐ข๐๐ค๐ฌ ๐๐จ๐ซ ๐๐จ๐ฆ๐ฉ๐ฅ๐๐ฑ ๐๐๐ง๐๐ฌ๐๐๐ฉ๐๐ฌ: Conversely, if your organization deals with a complex data landscape characterized by unpredictable sources and schemas, Databricksโwith its schema-on-read techniqueโmay be more advantageous.